The first two-dimensional (2D) or single-layer material to be isolated in a lab, graphene was initially “manufactured” prosaically in 2004. Andre Geim and Konstantin Novoselov, two physicists from the University of Manchester in the UK, obtained the crystalline solid by using sticky tape to exfoliate graphite. Both materials are composed solely of carbon atoms. But the geometry of the carbons in graphene is different to graphite—and this gives it certain unique properties. In graphene, they form a single sheet of atoms in a hexagonal mesh, like a honeycomb. In graphite, there are several layers of graphene separate from each other.
Since graphene was first discovered in this almost banal way, the methods used to search for other 2D materials with unique properties have become more sophisticated. Currently, one of the most promising ways of searching for materials of interest formed exclusively by one layer of atoms, which are often composed of more than one chemical element, is with artificial intelligence (AI), especially machine learning. This computational tool uses statistical models to predict the most likely characteristics of a 2D material, whether experimentally manufactured or purely theoretical.
The reverse path can also be taken with this approach. “We can use machine learning to search databases for two-dimensional materials more likely to exhibit one or more properties of interest to us,” explains Gustavo Dalpian, a physicist from the Federal University of ABC (UFABC) who is studying the topic with Adalberto Fazzio of the Brazilian Center for Research in Energy and Materials (CNPEM), with funding from FAPESP. “This is a relatively new area of research called materials informatics, which is allowing us to move towards using big data to process a large amount of information.”
Two recent studies by the UFABC group illustrate how this approach can generate knowledge of 2D materials, whose tiny scale on the order of nanometers and particular properties can lead to even greater advances for existing devices as well as the creation of new equipment. An article published in the journal ACS Applied Materials & interfaces in February indicates that machine learning is highly effective, with around 90% accuracy, at predicting whether or not a 2D material is magnetic. In another study, Dalpian and his colleagues are using a similar methodology to identify structures formed by a single layer of atoms that tend to have a specific spin texture—an intrinsic quantum property of subatomic particles, such as electrons, associated with angular momentum. A paper was published in Scientific Data describing the research on April 29.
Two-dimensional materials are formed by a single layer of atoms
Machine learning systems are taught to recognize different patterns associated with conditions or characteristics within a large dataset. Samples with one of these expected signatures are separated and classified. “This is a knowledge discovery process,” says Osvaldo Novais de Oliveira Júnior, a physicist from the São Carlos Institute of Physics (IFSC) at the University of São Paulo (USP) who did not participate in the studies by Dalpian’s team. “Humans make inferences from a small amount of data.”
In oncology, for example, a machine-learning algorithm can be programmed to recognize the key visual features that distinguish certain types of skin cancer, such as shape and color, from a benign mark. The system examines images of skin lesions and separates those with the determined characteristics—and which therefore have a high chance of being a tumor—from those that do not fit the profile.
The same logic can be used in the search for molecules or compounds with specific attributes. Just teach the machine-learning algorithm to recognize patterns associated with magnetism in 2D materials, the subject of the first study by the UFABC group, and the system is ready. Magnetism is a fundamental property when constructing information-storage devices, such as computer hard drives. The use of 2D materials with this property could lead to an even greater reduction in the size of such devices.
“The problem is that the typical magnetic signature of a 2D crystal is unknown,” says physicist Carlos Mera, who is doing a postdoctoral fellowship with Dalpian’s team and was a coauthor of both articles. “Until about five years ago, it was thought that the internal geometry of 2D materials generated instabilities that made them incompatible with magnetism.”
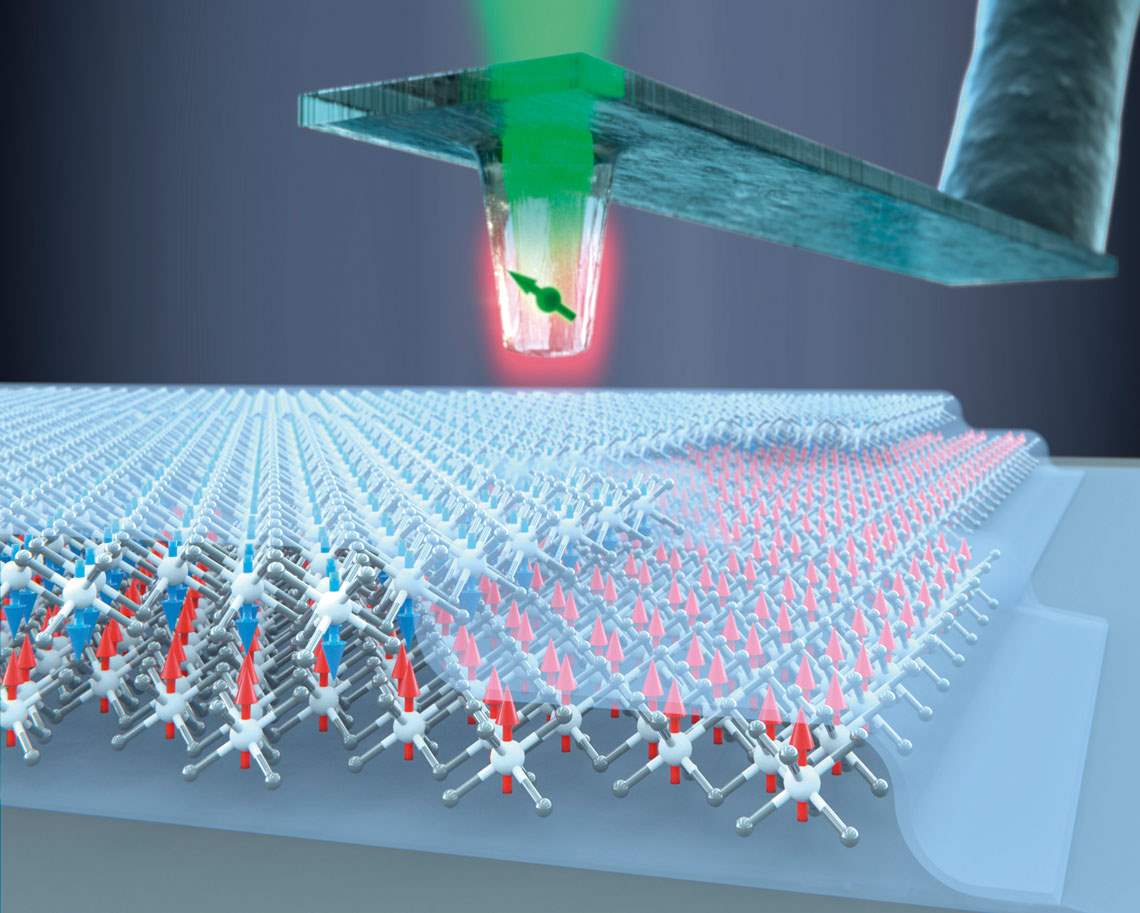
Department of Physics / University of BaselIllustration of a quantum sensor used to measure the magnetic properties of a two-dimensional material called chromium triiodide (CrI3)Department of Physics / University of Basel
In 2016, a group led by scientists from the Institute of Basic Science in Seoul, South Korea, measured antiferromagnetism, a type of magnetism, in films of nickel phosphorus trisulfide (NiPS3), a 2D material. The following year, a group from the University of California, Berkeley, observed ferromagnetism, another form of magnetism, in 2D chromium germanium telluride (Cr2Ge2Te6) crystals. Since then, magnetism has been observed in more 2D materials.
In response to this trend, the UFABC team decided to analyze the Technical University of Denmark’s Computational 2D Materials Database (C2DB). When the study began, the database contained records on some 3,400 materials (now 4,000). The objective of the research was to find out if there is a set of characteristics that could function as a strong indicator of magnetism in crystals formed by just one layer of atoms. In other words, whether there is a typical configuration associated with this property in 2D materials, much like the standard skin cancer diagnosis based on the shape and color of a mark on the skin.
The strategy was successful. By analyzing the characteristics of three key parameters of 2D materials, the machine-learning system was able to predict whether a crystal had a high probability of being magnetic with 85% accuracy. The program was even more precise, with 96% certainty, at estimating if a material is not magnetic. The parameters analyzed were the chemical composition, the crystal structure, and the strength of the spin-orbit interaction (a quantum property).
The study was based on the premise that 2D materials composed of atoms of a transition metal (chemical elements from groups 3 to 12 of the periodic table), with hexagonal (such as graphene), square, or triangular crystal structures and strong spin-orbit interaction are highly likely to be magnetic. According to the predictions of the machine-learning system, 478 materials in the database are magnetic, of which 373 are ferromagnetic and 105 antiferromagnetic.
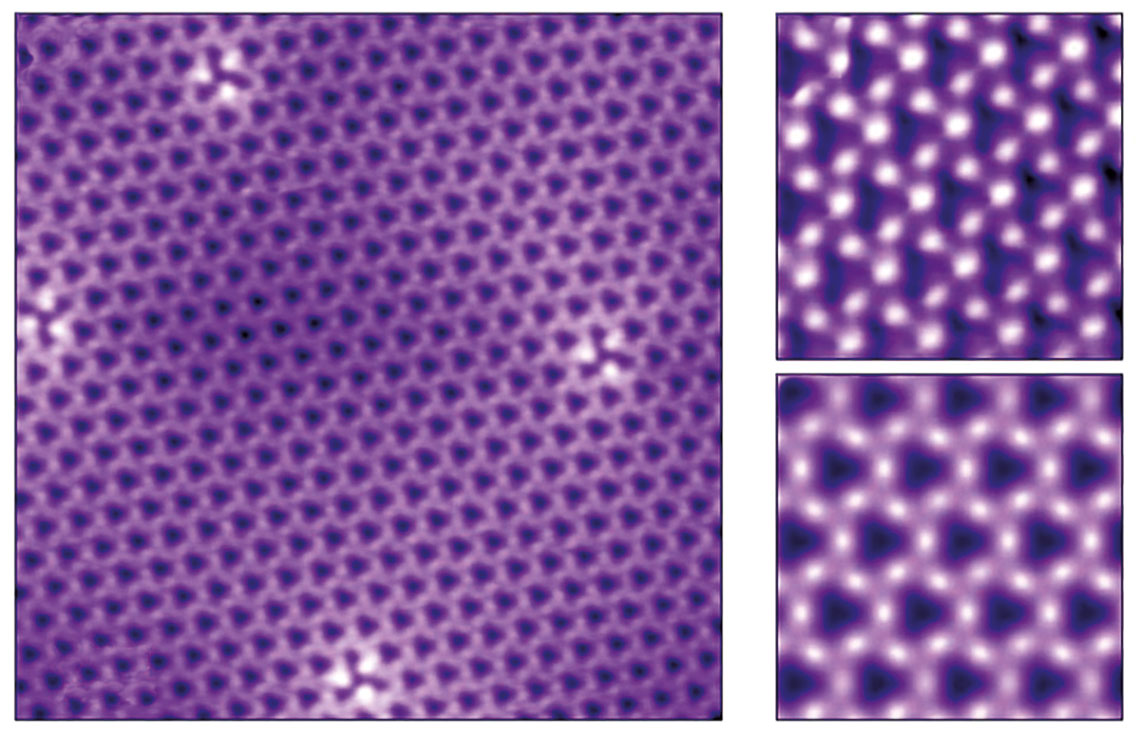
Zhenqi Hao, 2018 Science BulletinImage of the hexagonal structure of the material Cr2Ge2Te6Zhenqi Hao, 2018 Science Bulletin
The C2DB database actually included information on the magnetism of each material before the Brazilian researchers started their work. Knowing this information was essential to determining the accuracy of the machine-learning technique and validating the method. “What we did was to find out whether a small number of properties are capable of functioning as filters to allow us to predict—with the help of big data techniques—whether a 2D material is likely to be magnetic or not,” explains Dalpian. “We were able to achieve this goal and we can even tell whether the material tends to be ferromagnetic or antiferromagnetic.”
It is therefore possible, when searching for new magnetic 2D materials, to immediately discard any of crystals that the system determines as having a very low chance of being magnetic. Scientists thus waste less time and effort on unknown compounds that are unlikely to be magnetic and can focus on more promising materials.
In the second study, the researchers used the C2DB database to search for materials that had a quantum effect associated with an electron configuration known as spin splitting. According to the electrons’ spin state (or angular momentum), whether they point up or down, the atoms of a material exhibit different configurations that in some cases can change their energy levels. There are four known variations of spin splitting: Zeeman, Rashba, Dreshelhaus, and higher order.
“We calculated that 436 materials in the database likely present some form of spin splitting. For each crystal, we determined which kind of this effect it is likely to present,” says Elton Ogoshi, a materials engineer doing a doctorate at UFABC and coauthor of the study. In theory, the spin control of 2D materials can serve as the basis of spintronics, a form of computation based on manipulating this quantum property to store and process information.
In addition to machine-learning algorithms, this second article by the group was also based on the use of a statistical technique known as Bayesian inference, which is widely used in AI to update the probability of a hypothesis occurring as more evidence or information becomes available. “Bayesian inference is a way of classifying data,” says Oliveira Júnior. It is used to choose the most likely hypothesis. While it increases the chance of success, it does not fully eliminate errors.
Project
Material interfaces: Electronic, magnetic, structural, and transport properties (nº 17/02317-2); Grant Mechanism Thematic Project; Principal Investigator Adalberto Fazzio (CNPEM); Investment R$7,540,853.49
Scientific articles
NASCIMENTO, G.M. et al. High-throughput inverse design and Bayesian optimization of functionalities: spin splitting in two-dimensional compounds. Scientific Data. On-line. apr. 29, 2022.
ACOSTA, M.A. et al. Machine Learning Study of the Magnetic Ordering in 2D Materials. ACS Applied Materials & Interfaces. On-line. feb. 9, 2022