In May of this year, the US food and drug agency (FDA) granted California-based company VitalConnect emergency authorization to use its VitalPatch biosensor to monitor heart problems caused by COVID-19 or drugs used to treat the disease. The wearable device, which looks like a bandage glued to the chest, is capable of remotely monitoring 22 different types of arrhythmias, in addition to other physical parameters, such as heart and respiratory rate, temperature, and body posture.
The potential for remote health monitoring and assessment is important given the need for social distancing during the pandemic and the overcrowding seen in many hospitals. This demand has accentuated a growing trend of recent years. An increasing number of companies and academic research groups, both in Brazil and abroad, are studying and developing cardiology devices that use artificial intelligence (AI). Another indicator of this growth is the rising number of scientific articles published on the topic in the last decade (see infographic).
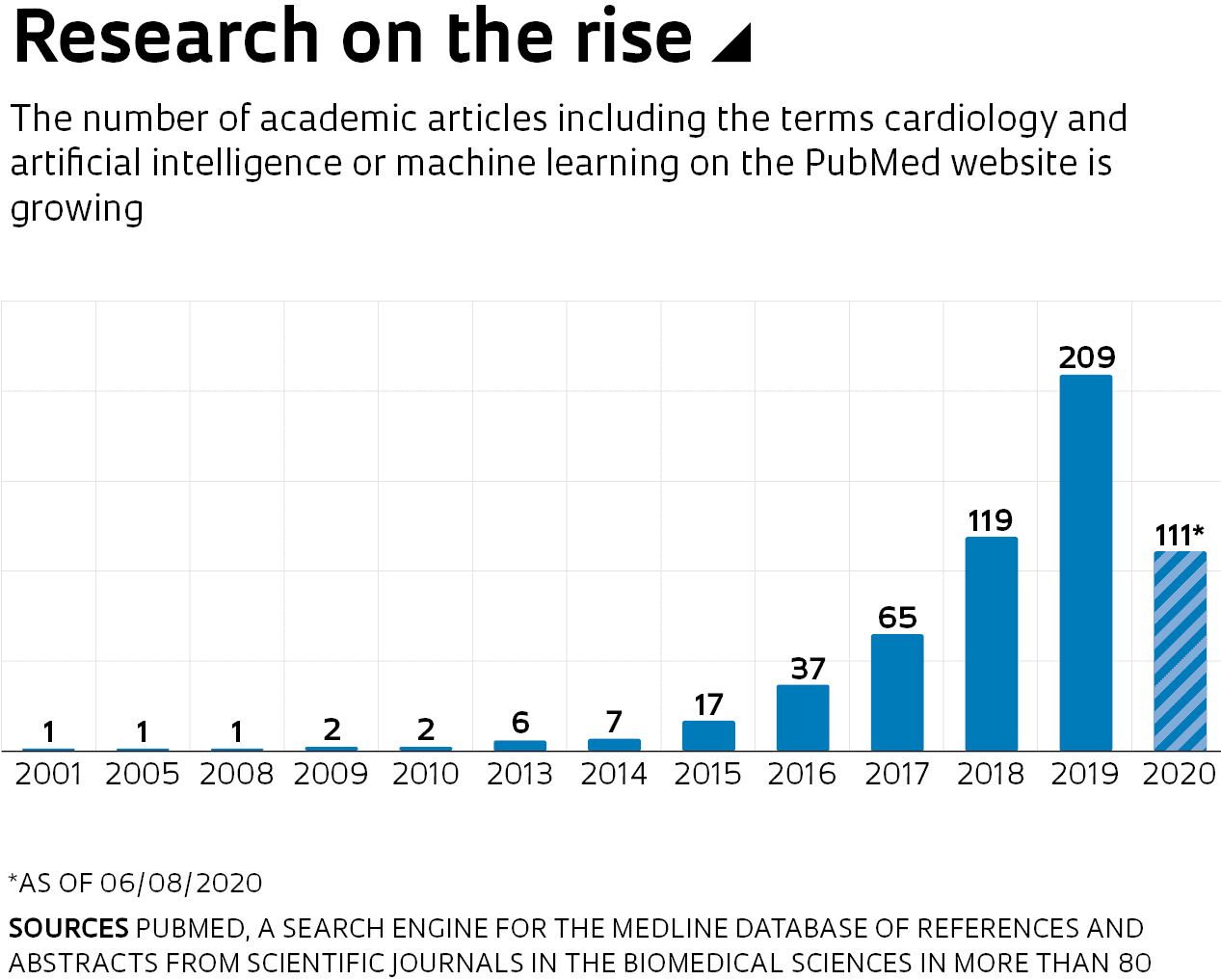
The year 2020 began with a new milestone in this regard. In February, Caption Health, based in Silicon Valley, was the first company to receive FDA authorization for an AI imaging exam. The system, called Caption AI, enables healthcare professionals to perform echocardiograms even if they have no experience in cardiology. The software helps users capture images of diagnostic quality.
Artificial intelligence techniques, including data mining and machine learning, can also help analyze echocardiogram images. Ultromics, initially founded at the University of Oxford, UK, already supplies its EchoGo system to the British National Health Service (NHS) and in late 2019 obtained authorization from the FDA to enter the US market. According to the company, EchoGo can identify abnormalities in echocardiogram pictures within a matter of minutes. The software was developed by analyzing 120,000 images from exams conducted by the University of Oxford. The echocardiogram images are automatically sent to the cloud-based system for analysis, and a report is then sent to the physician.
“The computer system is designed to support doctors, not to replace them,” emphasizes computer scientist Fátima Nunes, from the School of Arts, Sciences, and Humanities (EACH) at the University of São Paulo (USP). Nunes is supervising an image-processing project by master’s student Matheus Alberto de Oliveira Ribeiro, funded by FAPESP. Cardiologist Carlos Rochitte, from the Heart Institute (InCor) at the USP Medical School (FM-USP) and coordinator of the Magnetic Resonance and Cardiovascular Tomography Service at Hospital do Coração (HCor) in São Paulo, is also contributing to the research.
Rochitte provides MRI images that are used to teach the software—through machine learning—to recognize patterns and thus diagnose abnormalities. The cardiologist explains that as the strongest of the heart’s four chambers, the left ventricle is the organ’s driving force. “After a severe heart attack, damage to the myocardium [heart muscle] causes the ventricle to change shape. We call this ventricular remodeling,” he says. These changes can help make diagnoses. A heart that loses its characteristic shape, which vaguely resembles a cone, and becomes more spherical, for example, may indicate dilated cardiomyopathy.
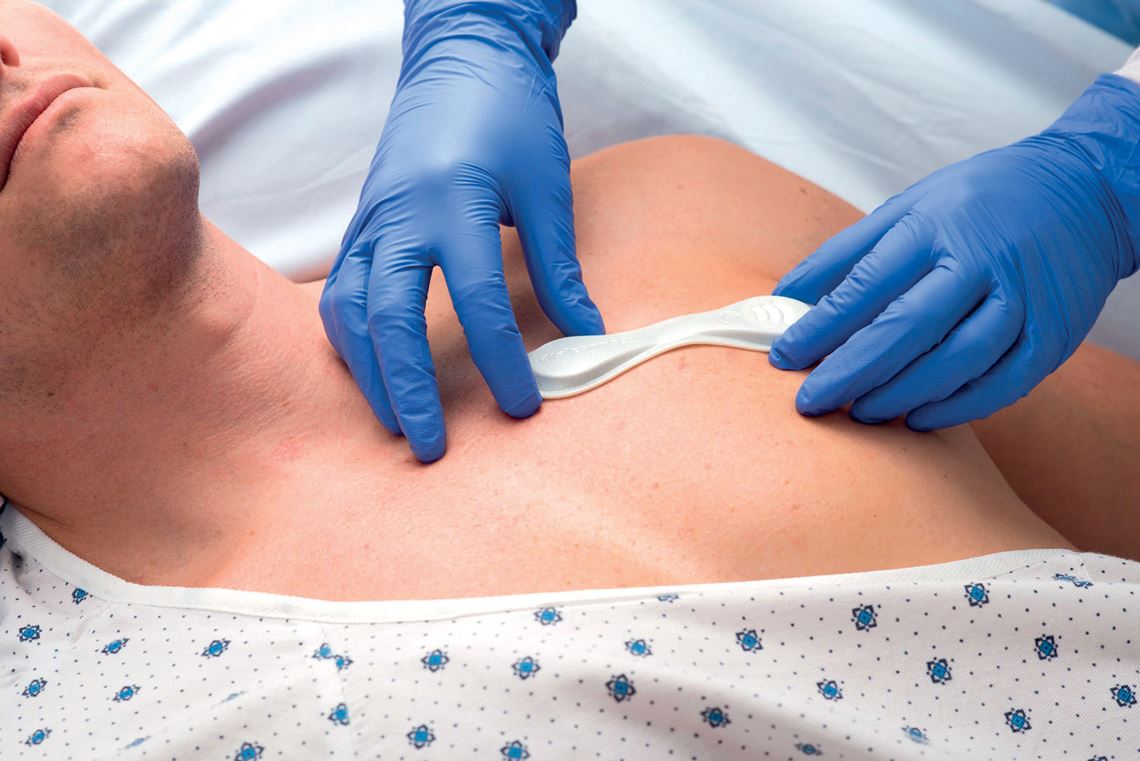
VitalConnect
The VitalPatch wearable biosensor monitors heart problems caused by COVID-19VitalConnectAn MRI scan can analyze the shape of the left ventricle. The procedure takes multiple two-dimensional slices and then puts them together, like a pile of coins. “About 200 to 300 images are needed to fully analyze the left ventricle, and the physician has to look at them one by one,” says Nunes. “As well as being time-consuming, looking at so many images can lead to fatigue and an increased risk of human error.”
Computer software already exists for two-dimensional analysis of the left ventricle. Ribeiro’s proposal is innovative in that it looks at the exam from a 3D perspective. “We create a three-dimensional object. Instead of analyzing each image one by one, we can look at the ventricle as a whole and calculate certain metrics to help reach a diagnosis. For example, we can estimate the size of the heart and the volume of blood it is pumping, which would not be possible with the traditional 2D exam,” he explains. Rochitte points out that “there are other similar initiatives under development, but the approach taken by this research is unique—the proposed three-dimensional model is unprecedented.” A working prototype of the tool, which will be made available to InCor, is almost ready.
Most AI cardiology projects in Brazil are still in the academic stages, but there are a number of commercial products on sale. The Fleury Group uses AI to diagnose cardiovascular diseases with a focus on two approaches: detecting intracranial hemorrhages caused by strokes, and pulmonary embolisms, a condition in which one or more arteries in the lungs become blocked by blood clots.
In 2019, its study on detecting intracranial hemorrhages was recognized at the Radiological Society of North America (RSNA) Conference, the largest radiology and diagnostic imaging event in the world. The institution already uses a tool for detecting pulmonary embolisms, says radiologist Gustavo Meirelles, head of Radiology, Strategy, and Innovation at the Fleury Group.
The device was developed together with Israeli startup Aidoc, and according to Meirelles, reduces diagnosis time from 3 hours to about 20 minutes. “The first time we used it, the exam result was ready before the patient even left the hospital. We were able to begin treatment there and then,” says the doctor. “Early diagnoses lead to better patient outcomes, reducing the length of hospital stays and lowering mortality rates.” Meirelles stresses that these new computer tools have only been developed thanks to a solid set of data used to train them.
The computer system is designed to support doctors, not to replace them, emphasizes computer scientist Fátima Nunes, from USP
Physiologist José Eduardo Krieger, director of the Genetics and Molecular Cardiology Laboratory at InCor and a professor at FM-USP’s Department of Cardiopneumology, believes data volume and quality is the foundation of any advance in the field of AI. The InCor studies actually began in the area of big data, through the institution’s IT services. “InCor has been paperless [all of its processes are digital] for over 10 years,” says Krieger. “The electronic medical records system was created by a team led by engineer Marco Antonio Gutierrez, director of Bioinformatics at InCor, through several projects, some of which were funded by FAPESP,” he recalls.
Today, the system has about 1.3 million registered patients. More than 30 hospitals in São Paulo use it, giving researchers access to 10 million records—all of which remain anonymous. For Krieger, it is a precious resource: “Using these computer systems, we were able to advance to the AI tools that we are developing today.”
InCor is the headquarters of the Brazilian National Institute of Science and Technology in Medicine Assisted by Scientific Computing (INCT-MACC), established in 2008 to consolidate technological development and training, funded by FAPESP and the Brazilian National Council for Scientific and Technological Development (CNPq). The institute coordinates 31 laboratories in 11 Brazilian states and another 17 based abroad, in seven different countries.
InCor is currently focusing its efforts on four major areas of study: image, signal, and language processing, and data integration in the “omic” sciences (genomics, proteomics, metabolomics, etc.). “These fields also involve partnerships with multinational technology companies, such as Canon and Foxconn,” says Krieger. Foxconn is a computer and smartphone manufacturer whose customers include Apple and Microsoft.
The most advanced research is in processing tomography and magnetic resonance images and electrocardiographic signals. In electrocardiogram analysis in particular, there has been significant progress: the creation of AI algorithms that can diagnose problems simply by examining the resulting graph. “Electrocardiograms are cheap and widely used, but interpreting the results can be more difficult than you might think,” says Krieger. “With the InCor tool, physicians can take a picture of the exam output and send it to the system for assessment, regardless of the equipment used.”

Prediction of cardiovascular risk factors based on retinal fundus photographs and deep learning
Method developed by American scientists differentiates between healthy eyes and those with damaged blood vessels at the back of the eye, an indication of cardiovascular problems. The tool can also predict the age, gender, glycated hemoglobin level (an indicator of diabetes), and body mass index of the patient with a low margin of error, as well as whether they are a smoker or not.Prediction of cardiovascular risk factors based on retinal fundus photographs and deep learningThe challenge of this new line of research, according to Krieger, is the development of risk prediction algorithms that take into account the particular characteristics of every individual. “We attempt to integrate data from the patient’s entire clinical history, including genetic markers,” reports the researcher, who supervises graduate phenotyping and genotyping studies on cardiovascular diseases at USP.
Some projects are already advancing in this field. In the US, scientists at startup Verily Life Sciences, Google Research, and Stanford University have created a technique for predicting cardiovascular risk from retinal examinations. They trained a machine-learning system with retinal images of 284,000 patients from the UK Biobank and EyePACS databases. Based on these images, the system learned to distinguish between healthy eyes and those with damaged blood vessels visible at the back of the eye, an indication of cardiovascular problems.
The group’s objective was to determine whether signs of cardiovascular risk could be identified quickly, cheaply, and noninvasively in an outpatient setting. The results exceeded expectations: by comparing images, the algorithm was also able to determine the age, gender, glycated hemoglobin level (an indicator of diabetes), and body mass index of the patient with a low margin of error, as well as whether they were a smoker or not. “We show that deep learning can extract new knowledge from retinal images,” highlighted the study authors. “With these models, we predicted cardiovascular risk factors not previously thought to be present or quantifiable in retinal images.”
Deep learning is the latest generation of machine learning. It is a computational method based on an artificial neural network with multiple layers—which is why it is called deep learning. With this approach, instead of being manually programmed to perform a specific task, the computer uses generic algorithms to identify patterns in images, text, or signals.
The same technology is being used by Projeto CODE (Clinical Outcomes in Digital Electrocardiology), a team formed by researchers from the Telehealth Center of the Hospital das Clínicas (HC) at the Federal University of Minas Gerais (UFMG). With funding from the Minas Gerais Research Support Foundation (FAPEMIG) and collaboration with the universities of Glasgow in Scotland, and Uppsala in Sweden, the group developed an automated tool capable of reading electrocardiograms and diagnosing heart disease.
Predictive medicine research at UNICAMP could save the Brazilian Public Health System (SUS) R$50 million per year
The project involves physicians, engineers, and computer scientists. “The secret is to assemble a truly multidisciplinary team,” says cardiologist Antonio Luiz Pinho Ribeiro, leader of the group and head of the Telehealth Center and the Minas Gerais Teleassistance Network, which comprises seven public universities from the state. The result of the study, which cross-referenced 2.4 million digital electrocardiograms taken between 2010 and 2017 with data from Brazil’s National Mortality Information System, was published in the journal Nature Communications in April. The software was able to recognize patterns and identify six different types of electrocardiographic changes with equal or greater accuracy than medical residents and students.
It also has another special feature. “We used a neural network to predict the patient’s age based solely on the electrocardiogram. This electrocardiographic age could be a useful indicator of cardiovascular health,” explains engineer Antônio Horta Ribeiro, who is part of the team. Early results suggest that when the electrocardiographic age predicted by the algorithm is older than the person’s actual age, there is a greater risk of mortality in all age groups.
Ribeiro is an AI specialist, and believes that research involving neural networks could provide medicine with new diagnostic perspectives. The software, previously trained to recognize patterns, is now capable of spotting anomalies that go unnoticed by humans. “It can identify correlations that have not yet been found by medicine,” he says. The challenge is to try to identify the paths the neural networks took to establish these correlations.
A predictive medicine study at the University of Campinas (UNICAMP) is hoping to create savings of up to R$50 million per year for the Brazilian Public Health System (SUS). Conducted by the Aterolab Laboratory at the university’s School of Medical Sciences (FCM), the project aims to identify patients with chronic coronary heart disease who are at greater risk of suffering adverse clinical events within the next 12 months. The research, overseen by cardiologist Andrei Sposito, who runs the Aterolab, was recognized by the Brazilian Society of Cardiology and the European Conference on Innovation.
“In the first year after a heart attack, one in five patients will suffer another heart attack or sudden death. Many attempts have already been made to identify patients most at risk of these complications,” says the cardiologist. Sposito points out that cardiovascular risk factors have been known for many years, but they cannot be calculated arithmetically. “There are simultaneous actions related to individual characteristics. Having two risk factors does not mean someone is at twice as much risk. It’s not that simple,” he explains.
According to the cardiologist, the first mathematical models for these variables were created in the 1970s, but even today the results are not highly effective. Artificial intelligence, he says, has changed the game. In his research, AI algorithms were able to predict 92% of the clinical events that a patient may experience within the following year. Based on this data, explains Sposito, the most vulnerable patients can be closely monitored, which could prevent deaths and reduce the need for readmissions to hospital, surgery, and expensive treatments. “These are important advances resulting from research into AI for disorders of the heart,” concludes the expert.
Projects
1. Automatic segmentation of the left ventricle in cardiac magnetic resonance imaging (no. 19/22116-7); Grant Mechanism Master’s (Msc) Fellowship; Supervisor Fátima de Lourdes dos Santos Nunes Marques (USP); Beneficiary Matheus Alberto de Oliveira Ribeiro; Investment R$39,863.34.
2. INCT 2014: Assisted Medicine by Scientific Computing (INCT-MACC) (no. 14/50889-7); Grant Mechanism Thematic Project; Principal Investigator José Eduardo Krieger (USP); Investment R$3,204,512.68.
3. Artificial Intelligence Center (no. 19/07665-4); Grant Mechanism Engineering Research Centers; Principal Investigator Fabio Gagliardi Cozman (IBM); Investment R$4,134,883.90.
Scientific articles
RIBEIRO, H. A. et al. Automatic diagnosis of the 12-lead ECG using a deep neural network. Nature Communications. Apr. 9, 2020.
POPLIN, R. et al. Prediction of cardiovascular risk factors from retinal fundus photographs via deep learning. Nature Communications. Feb. 19, 2018.