Vehicles with advanced self-driving systems are already circulating in cities in the USA, Asia, and Europe. In China, technology company Baidu, owner of ride-hailing app Apollo Go, and startup AutoX, linked to the Alibaba conglomerate, offer experimental robotaxi services in some of the country’s biggest cities, including Beijing, Shanghai, and Shenzhen. Currently available autonomous vehicles, however, can only be used in limited scenarios: a safety driver must be in the car to take control in emergency situations; or they must drive below certain speeds—usually around 40 to 50 kilometers per hour (km/h)—on predetermined circuits where obstacles and risks have already been mapped.
As yet, there are no fully automated cars capable of traveling safely on any route without human intervention. “Autonomous cars that interact with city infrastructure, reducing traffic and increasing comfort and safety for passengers and pedestrians are the future of mobility, but this is a transformation that will still take 20 or 30 years to happen,” says Fabio Kon from the Computer Science Department of the Mathematics and Statistics Institute (IME) at the University of São Paulo (USP).
Vehicles are classified into five levels of autonomy (see infographic), according to a scale created by SAE International, an organization representing scientists and specialists in automotive engineering. These cars operate using a set of systems, including sensors to observe their surroundings and capture information on the presence of pedestrians, cyclists, objects, and other cars on the road and nearby, and artificial intelligence (AI) to interpret this information and transform it into actions such as speed control, braking, and lane changes. They also need electronic navigators, such as a Global Positioning System (GPS), to plan and follow routes.
“The systems needed for autonomous vehicles already exist, but we still need to improve the quality of information captured by sensors and the ability of AI to interpret this information,” says Fernando Osório, from the Institute of Mathematical Sciences and Computing (ICMC) at USP, São Carlos campus. Public and private research centers in many countries are working on solutions to these challenges.
One of the biggest ongoing projects in Brazil is the Integrated Development of Driver and Environment Assisted Safety Functions for Autonomous Vehicles (SegurAuto), a working group formed in 2021. The team is composed of researchers from USP, the Federal Technological University of Paraná (UTFPR), the federal universities of Brasília (UnB) and Pernambuco (UFPE), and the companies BMW, Stellantis (formerlu Fiat Chrysler), Renault, Mercedes-Benz, Bosch, AVL, DAF Caminhões, and Vector Informatik.
“The purpose of SegurAuto is to develop advanced driver-assistance systems that can be gradually incorporated into vehicles until they become fully autonomous,” explains Evandro Leonardo Silva Teixeira, from the automotive engineering course at UnB. Examples of such innovations already adopted by automakers include electronic stability control, automated parking systems, and adaptive cruise control, which allows drivers to set a cruising speed and minimum distance from the car in front.
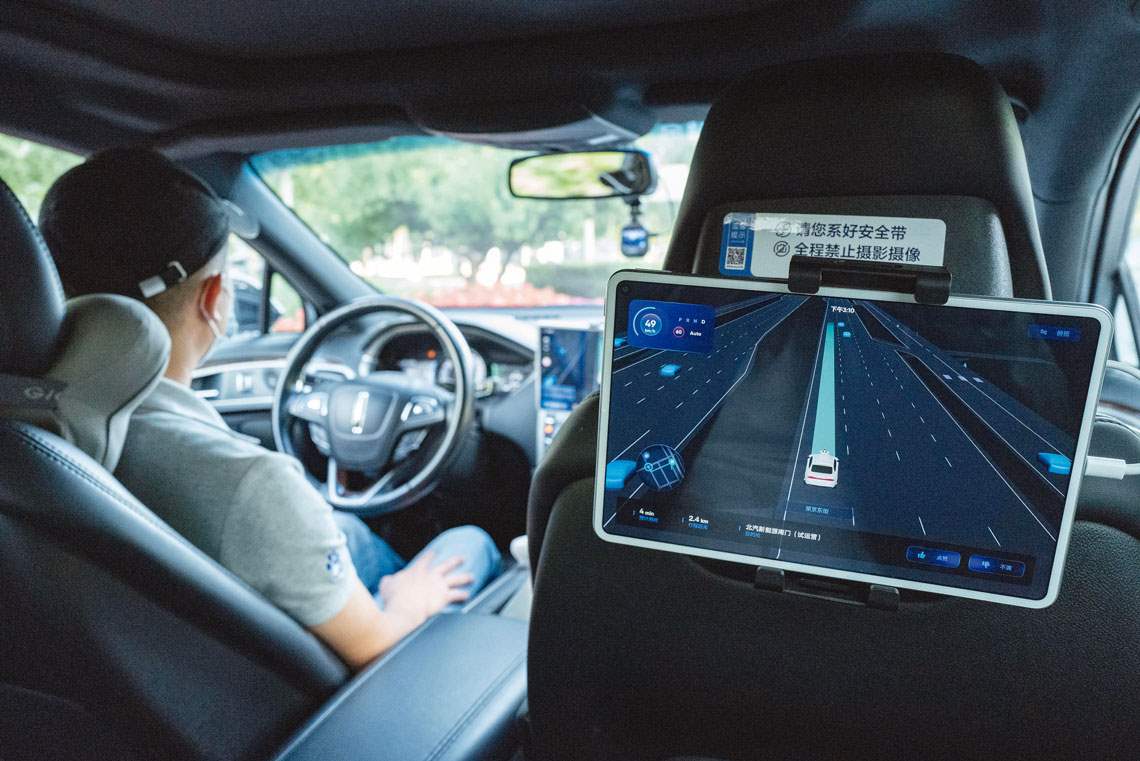
Robotaxi in the Chinese city of Beijing: limited autonomyHou Yu / China News Service via Getty Images
Combined sensors
One project the SegurAuto team is working on is the combination of two types of sensors to read the vehicle’s surrounding environment: radars, which use radio waves to detect the presence of elements; and computer vision, which uses video cameras. According to Osório, head of the USP São Carlos group at SegurAuto, radar is capable of detecting unexpected obstacles in most situations. “If someone walks in front of a truck, for example, the radar promptly detects them and the vehicle immediately activates the brakes,” he explains. However, it does not always capture nuances and can only give an approximate idea of the characteristics of an obstacle.
Computer vision is more accurate. As well as identifying and detailing obstacles and objects near the road, it can be used to read traffic signs and identify pedestrian crossings. The problem is that video camera images are affected by adverse situations such as fog, rain, dust, the headlights of oncoming vehicles, and lights on emergency vehicles.
Tesla cars, which only use computer vision, offer an example of the system’s limitations. They have been involved in more than a dozen accidents, in several of which the sensors were unable to identify the lights of emergency vehicles stopped in the road, resulting in collisions with first responders.
“No single sensor alone is fully efficient. Combining different technologies is the best solution,” says Osório. Even lidar (light detection and ranging), the most effective sensor according to the scientist, which uses laser beams to measure the distance of objects and map the environment, is limited by its short range of 150 meters (m), meaning it has to be used together with other sensors.
Lidar also requires high-precision mechanisms and the support of high-performance processing units, resulting in operating costs that most automakers consider prohibitive. The biggest technology enthusiast in the self-driving car industry is Waymo, part of the Alphabet group—the parent company of Google. It stopped selling lidar solutions to other companies in 2021 and is now working on a new generation that is more efficient and cheaper.
The combination of radars and computer vision proposed by the SegurAuto team has already demonstrated its operational effectiveness, says Osório, but the performance of each of the sensors individually needs to be improved. “We need to see farther and earlier,” he points out. Overtaking at 80 km/h requires anticipating situations 1 km away. “A standard radar has a range of 300 m. A video camera can see farther, but the information obtained is inaccurate. It requires major data-processing capacity and it takes too much time to receive the results”, describes the researcher.
Detecting distant obstacles is just the first step in the process. The system also has to determine which direction the object is moving in, the speed it is moving, and whether or not it represents a collision risk. Artificial intelligence is needed to improve the ability to interpret these kinds of scenarios.
Advances in telecommunications and the internet of things (IoT) could help improve vehicle safety. It is possible, for example, to anticipate hazards, such as two cars arriving at an intersection where the field of vision is limited at the same time. “Communication between vehicles and speed cameras, pedestrians’ cell phones, or other vehicles can generate information in advance,” says computer scientist Abel Guilhermino da Silva Filho, head of the Vehicular Innovation Laboratory (LIVE) at UFPE’s Informatics Center.
The UFPE team at SeguAuto is studying the Vehicle to Everything (V2X) and Cellular Vehicle to Everything (C-V2X) communication networks, which allow vehicles to exchange data with other cars, pedestrians, and urban infrastructure such as traffic lights, speed cameras, and CCTV.
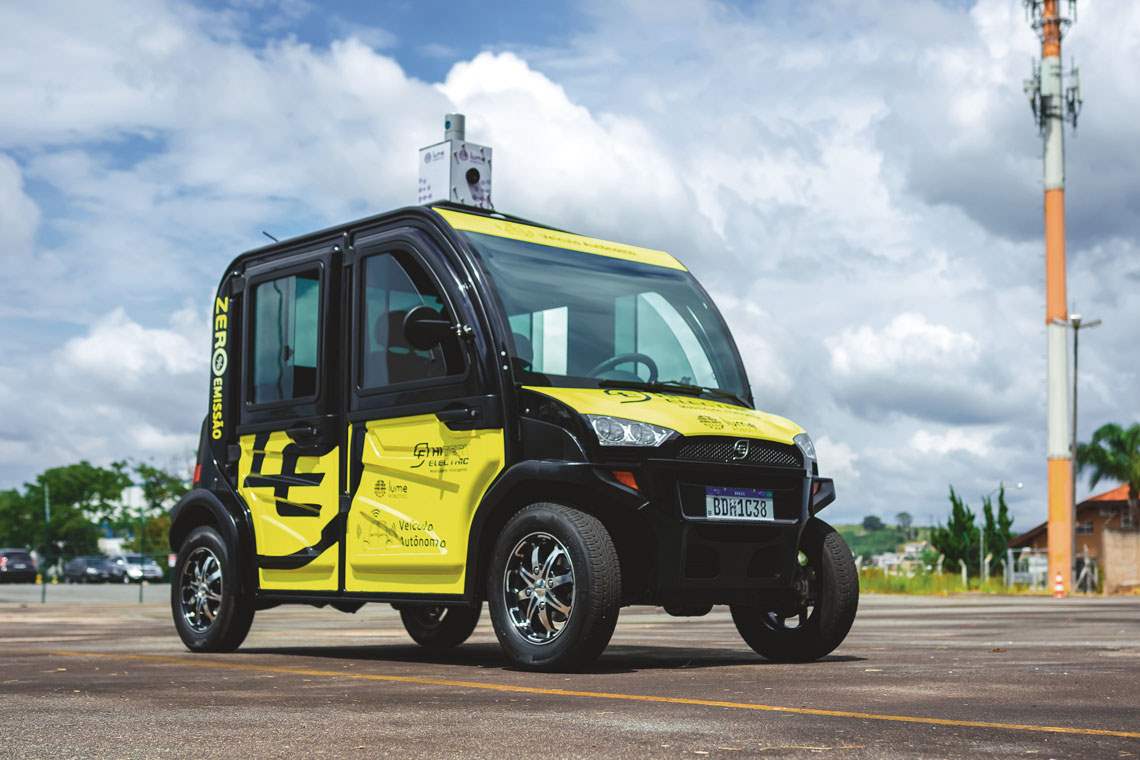
e.coTech 4: Brazil’s first commercial self-driving car, launched two years agoLUME
The group, led by Guilhermino, carried out a case study on convoys—commonly used in cargo transport—in which the lead vehicle makes decisions such as when to accelerate, brake, stop, or change route, and transmits them to the other vehicles behind. The lead vehicle relaying information to the rest of the convoy improves operational safety and fuel economy. It also means that only the lead vehicle needs a person on board to intervene in emergency situations. “We are establishing the best communication protocol, the time frame within which intervehicular communication needs to occur, and the amount of time needed to react safely,” the researcher explains.
Teams from UnB, led by Teixeira, and USP’s Polytechnic School (Poli-USP), led by physicist João Francisco Justo Filho, are in charge of developing software to convert the information collected by the sensors into decisions and actions taken by the vehicles. The UnB researchers are looking for ways to control steering and predictive braking, while those at Poli-USP are working on engine and braking systems.
Another national research group is based at Brazil’s National Institute of Science and Technology for Cooperative Autonomous Systems (INSAC), which is funded by FAPESP and has been contributing to the field since 2009, when it was called INCT SEC (Critical Embedded Systems).
The group helped develop the Intelligent Robotic Car for Autonomous Navigation (CARINA), the first Brazilian self-driving vehicle to navigate the streets of a Brazilian city (São Carlos in 2013), the first autonomous truck in Latin America, and the first Brazilian driverless agricultural machine (see Pesquisa FAPESP issues 213, 235, and 271, respectively). All were developed by the Mobile Robotics Lab at ICMC, USP. The Intelligent Systems Laboratory (EESC) at USP’s São Carlos School of Engineering also contributed to the development of CARINA and the autonomous truck.
In 2019, a team of six ICMC graduate students coordinated by professors Denis Wolf and Osório designed a virtual autonomous vehicle and won the Car Learning to Act (CARLA) Autonomous Driving Challenge, a competition run by automakers and autonomous driving technology developers. Sixty-nine teams from the most prestigious educational institutions in the world took part in the competition.
A team led by Valdir Grassi Junior, a mechanical engineer at InSAC and associate professor at the Department of Electrical Engineering and Computing (SEL) at EESC, USP, recently contributed to the field of computer vision through the development of a method for determining the depth of the environment based on images from a single camera mounted on a vehicle. The software analyzes an image and calculates the distance between the vehicle and surrounding objects. The results of the study were published in the journal Robotics and Autonomous Systems in 2021.
“Like humans, computer vision traditionally uses two cameras to determine depth. When a human loses sight in one eye, their brain takes a while to adapt to monocular depth perception. An autonomous vehicle needs to be capable of driving safely if a camera fails, so we need to create algorithms trained for such a situation,” explains Grassi.
Marco Henrique Terra, an electrical engineer at SEL and coordinator at INSAC, says that negotiations are at an advanced stage with a truck manufacturer on the development of autonomous heavy vehicles for use in critical environments in agriculture and mining. “These are two major areas of interest for multinationals thinking about investing in Brazil,” says Terra.
In 2017, project IARA (Intelligent Autonomous Robotic Automobile) at the High-Performance Computing Laboratory (LCAD) of the Federal University of Espírito Santo (UFES) reached a milestone in Brazil when its vehicle carried out a 74 km assisted journey from the UFES campus in Vitória to the neighboring municipality of Guarapari. Some of the researchers involved in the project later formed the startup Lume Robotics in 2019. Two years ago, in partnership with Paraná-based electric vehicle manufacturer Hitech Electric, it launched Brazil’s first commercial autonomous car. The e.coTech 4 uses lidar, video cameras, and GPS, and is classified as level 4 on the SAE’s driving automation scale, meaning it can drive without human intervention on pre-mapped routes.
“The plan is to meet corporate transport demands, for getting around large industrial plants, for example,” says Rânik Guidolini, founding partner of Lume. An autonomous truck designed for industrial sites is expected to begin testing in 2022. “We have five pilot projects scheduled. We hope to sign the first contracts this year,” says Guidolini.
Avenida Paulista with no drivers
Study simulates São Paulo’s most famous road with lanes dedicated to fully autonomous vehicles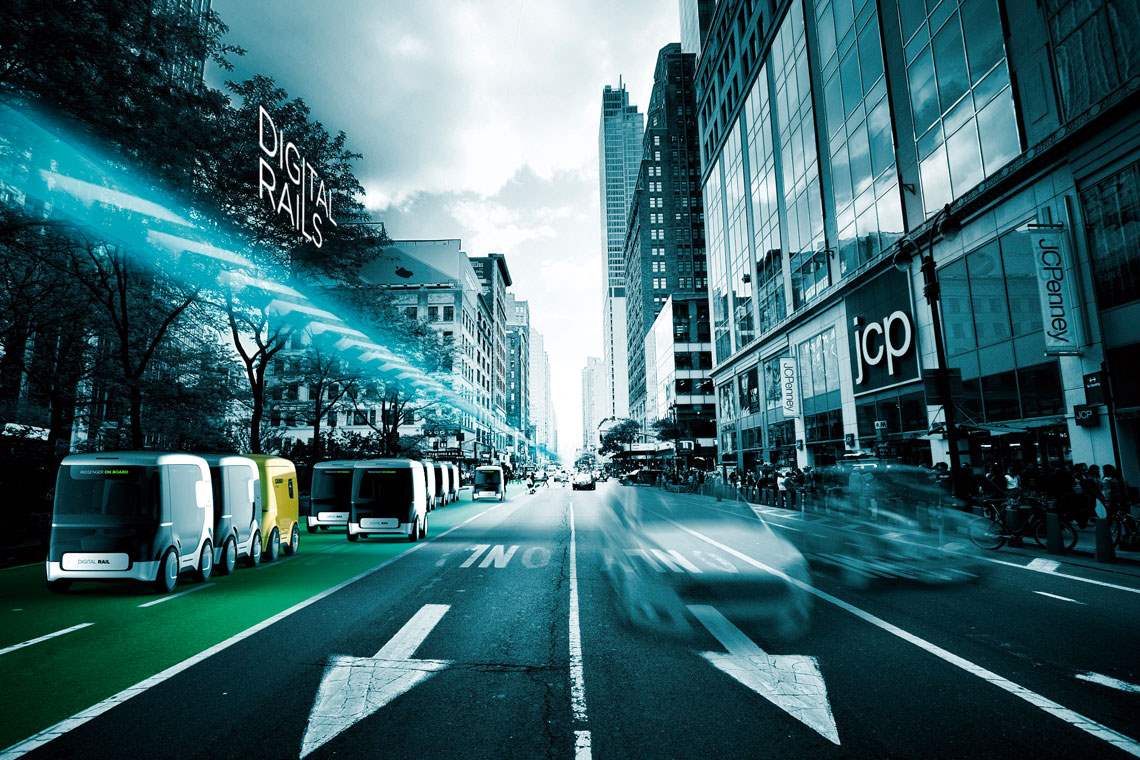
Illustrative image of a road with one lane dedicated to autonomous vehiclesQuesttonó Manyone
A prevailing expectation among engineers, traffic specialists, and consultants in the automotive industry is that self-driving vehicles will accelerate a trend that is already being seen in many European cities: a reduced interest in the private ownership of automobiles. This trend is the result of two forces. Firstly, the increasing level of technology and advanced automation systems is making vehicles more expensive, which means they are less affordable to much of the population. The second is the growing use of apps to hail vehicles on demand, allowing users to avoid spending large amounts of money on buying vehicles, maintaining them, and paying taxes and insurance.
“Cars will just become a type of infrastructure to be accessed on demand,” predicts Fabio Kon, a computer scientist from IME, USP. He believes the new situation will provide a range of benefits, such as improving urban mobility and safety, drastically reducing accidents, and releasing large urban spaces in prime areas currently used as parking lots.
A study carried out by Kon and his team at the National Institute of Future Science and Internet Technology for Smart Cities (InterSCity), funded by FAPESP, simulated what Avenida Paulista in the city of São Paulo might look like if it were used primarily by autonomous cars. The results were published in the scientific journal Simulation Modelling Practice and Theory in February last year.
Located at the intersection between the south, central, and west zones of São Paulo, Avenida Paulista has four lanes in each direction and is always congested at rush hour. In a future dominated by on-demand self-driving cars, just one lane would be enough to handle the same number of passengers in individual transport as the whole road does today.
“Crossing the avenue will be much faster. Platoons of autonomous vehicles can be formed and traffic lights can be controlled according to the locations of these convoys,” explains Kon. The researcher warns, however, that simply replacing private vehicles driven by humans with autonomous cars would only generate modest mobility benefits.
Accident liability
In Brazil, like in most countries, permission is needed from the traffic authorities to test a self-driving vehicle on public roads. In the West, only Germany since February and some US states have specific legislation authorizing autonomous vehicles to be used in public with human drivers on board. A problem still to be resolved, however—even in Germany and the USA—is liability in the event of an accident. Who is to blame: the vehicle owner, the safety driver who did not intervene in an emergency, the vehicle manufacturer, or the supplier of the technology at fault?
According to Edvaldo Simões da Fonseca Junior, an engineer from the Department of Transport Engineering at USP’s Polytechnic School, legislation on autonomous vehicles will likely follow the example set in civil aviation. In the event of an air accident, the responsibility falls firstly on the airline. With driverless cars, the owner would be responsible, which in the future would likely be a company providing urban mobility or cargo transport services.
After an investigation into the cause of the accident, liability could later be shifted onto whoever generated the failure: the service provider, the automaker, or the technology supplier. To support such a process, vehicles would have to be fitted with a data recording system similar to the black boxes found on planes. “It is also possible that in the future, traffic regulators will require autonomous vehicle manufacturers to include redundant sensors to reduce the risk of failures,” suggests Fonseca.
Projects
1. INCT 2014: Brazilian National Science and Technology Institute for autonomous cooperative systems applied to security and the environment (nº 14/50851-0); Grant Mechanism Thematic Project; Principal Investigator Marco Henrique Terra (USP); Investment R$3,882,876.
2. INCT 2014: The internet of the future (nº 14/50937-1); Grant Mechanism Thematic Project; Principal Investigator Fabio Kon (USP); Investment R$1,805,100.01.
3. The internet of the future applied to smart cities (nº 15/24485-9); Grant Mechanism Thematic Project; Principal Investigator Fabio Kon (USP); Investment R$1,561,650.22.
4. Project Carina – Intelligent robotic car for autonomous navigation (nº 11/10660-2); Grant Mechanism Regular Research Grant; Principal Investigator Denis Wolf (USP); Investment R$60,700.15.
5. Project Carina – Location and control (nº 13/24542-7); Grant Mechanism Regular Research Grant; Principal Investigator Denis Wolf (USP); Investment R$82,334.60.
6. National Institute of Science and Technology – Critical Embedded Systems (INCT-SEC) (nº 08/57870-9); Grant Mechanism Thematic Project; Principal Investigator José Carlos Maldonado (USP); Investment R$1,734,565.96.
Scientific articles
MENDES, R.Q. et al. On deep learning techniques to boost monocular depth estimation for autonomous navigation. Robotics and Autonomous Systems. v. 136, 103701. feb. 2021.
SANTANA, E.F.Z. et al. Transitioning to a driverless city: evaluating a hybrid system for autonomous and non-autonomous vehicles. Simulation Modelling Practice and Theory. v. 107, 102210. feb. 2021.
Republish